(~30 minute read)
—
TLDR
- KPIs or KRs are how strategic intent is translated into desired behavior and actions yet errors in this step are all too common even among seasoned managers
- The two most common errors are 1) Selecting the wrong KPI, 2) Selecting conflicting KPIs as part of the portfolio of top-level KPIs for a discrete initiative
- A common root-cause reason underpinning both these errors is a failure to understand and appreciate the cause-effect relationships underpinning a complex business system and delineating the associated metrics into leading vs. lagging indicators. Lagging indicators can serve as mile markers and finish lines for a journey, however, it is much more effective to assign managers of initiatives and business processes metrics that are actionable leading indicators. This makes diagnosis and rectification of efforts that are off track significantly easier
- Most real-world initiatives are complex endeavors that have more than one objective and therefore necessitate multiple KPIs. The challenge however is that when you have a portfolio of KPIs, there are likely to be metrics that incent conflicting behaviors. This creates tremendous confusion and derailment risk. The solution to this problem is to always have one single north-star metric for a critical initiative that you are trying to maximize or minimize and think of the remaining KPIs as constraints. The north star metric can and should evolve over time
- Here are three specific situations where we got this wrong in the journey from $150M-$1B at Alteryx with material consequences:
- Assuming that our focus on and success in optimizing one metric when launching our revamped partner / channel program would ultimately drive success in another more desired KPI for the company (i.e. failure to recognize cause-effect relationships in complex systems)
- Defining success for our cloud platform launch with both adoption and commercial-centric KPIs without designating one to be the north star. When conflict arose, each group prioritized optimizing the metric aligned with their individual incentives which ended up sub-optimizing the overall outcome for the company. All of the myriad of issues that arose could have been prevented had there been a single common explicit North Star KPI
- Investing in a post-sale services and success program with the intent to drive indirect revenue but operationalizing it as a direct revenue program which was fine initially but created massive issues later on (i.e. implications of taking shortcuts in operationalizing indirect value-driving initiatives as direct value efforts through oversimplified KPI selection)
—
Metrics are one of the most foundational components of modern managerial control systems for an enterprise. Anyone who has spent even a cursory amount of time in business knows that metrics are the lifeblood of an organization and are a mechanism by which we measure the health of an organization and assess whether an initiative, goal, or more broadly an org as a whole is on track.
Business metrics can go by different names (performance indicator, key performance indicator, key result, output etc.). Though the context, exact definition, and usage of each of these terms may vary from one organization to another, they are all ultimately trying to serve a similar purpose. Metrics are the manifestation of a strategy and its execution. They are a mechanism by which to measure and keep score of some reality so it can be reconciled with the original intent to determine if reality is shaping up in line with expectations or not. If it isn’t, metrics enable a manager to diagnose, intervene and course-correct an operation or initiative in a coherent manner so they can achieve their desired results.
The operative letter and word in the acronym KPI or KR is ‘K’ or “Key”. The reason is that, for all practical purposes, there are an infinite number of metrics that one could pick from for any given initiative. The idea behind KPIs or KRs is to elevate the most critical of these performance measures to the top. In data-driven organizations, metrics drive behavior (especially when tied to compensation) so the selection of the right KPIs is absolutely paramount to ensuring that managers are incenting the right behavior that accomplishes the overarching objectives they have outlined. This is a foundational and crucial skill for managers at all levels to develop and yet, in my experience, so many fail to get it right. I don’t believe this is due to managerial incompetence in most cases, rather it’s due to a lack of awareness of certain principles.
In this post, I will explore two of the most common errors I have seen managers make with KPIs and delve into why this tends to happen. I’ll then cover three specific examples of where we as a leadership team at Alteryx were guilty of falling victim to these traps and risked derailing important strategic initiatives. I will also discuss a set of guidelines and rules by which to think of and approach the selection and setting of KPIs
Two of the most common mistakes I’ve observed managers make with KPIs are:
- Picking the wrong KPIs for an initiative or business process
- Selecting multiple conflicting KPIs or Key Results for an initiative or business process
While it may sound trivial on the surface, the selection of sub-optimal KPIs is frequently cited as one of the most common reasons for why strategic efforts don’t produce their desired outcomes. The primary, root-cause reason I’ve observed for both of these mistakes (but especially for #1) is a failure to understand and distinguish between leading and lagging indicators. This in turn is driven by the lack of an accurate and comprehensive understanding of the cause-and-effect relationships that underpin a business process or system. For simple business processes, these relationships can often be very straightforward and self-evident, however, most initiatives and business processes are comprised of complex systems with multiple interactions and moving parts which makes teasing out these relationships a lot harder.
Here’s a more concrete example. If you think of a business process or initiative as a long and complex assembly line, then outcome indicators represent the intermediate and final outputs of the assembly line. The leading indicators are the levers and cranks at the start and various midpoints of the assembly line which if you adjust, will have an impact on various facets of the final output of the assembly line. Metrics like total revenue, profitability and share price are ultimate lagging indicators while metrics such as trials, number of reps-hired, pipeline and sev-1 bugs are leading indicators that influence the final outcome. While an organization or team might have an overall goal that is structured around a lagging indicator (e.g. revenue booked or share price), there typically isn’t a whole lot a manager can do on a given day or even via a discrete initiative to directly move a massively lagging indicator like share price by a material amount. Instead, they must focus their energies on moving the levers of the “machine” in a manner that positively impacts the overall outcome they want. What that means is that strategic initiatives and business processes should be heavily skewed towards having leading indicators as their primary KPIs with a solid understanding of the causal relationships between leading activities and their outcomes.
Said differently using a practical example, a Go-to-Market leader of a scaled enterprise can’t show up to work on a given day and directly increase revenue by sheer force of will. What they must do instead is understand what the primary levers of growth are for the business (or their segment), identify which of those levers are controllable and to what degree and then establish business processes and initiatives oriented to high-leverage activities around those controllable inputs. These controllable activities should form the metrics that the sales managers should structure their processes and cadences around and what they should hold their subordinates accountable to. If at the end of a given period (week, month, quarter etc.), the manager does not achieve their OUTPUT metric (e.g. monthly revenue bookings), then at least one of the following must be true:
- Their team did not hit the designated target on the input/activity metric with a high enough quality bar (e.g. the goal for the number of new software trials initiated in a given period wasn’t met)
- The assumptions underpinning the magnitude of the relationship between inputs and outputs was incorrect resulting in a wrong target being set (e.g. a conversation rate of trials to closed deals of X was assumed whereas in reality, it should have been Y)
- The fundamental assumption of a causal relationship between input and outputs was flawed to begin with and therefore the activity selected wasn’t the right lever and activity to pursue in the first place (e.g. trials are not the primary driver of customer sign-ups and bookings, they are correlated with some other customer behavior that is the real causal driver of new customer sign-ups)
There can be several reasons for why any of these scenarios might occur. A common one I’ve observed is a lack of access to clean, high-quality and actionable data in a timely manner for managers to base their understanding of reality and their decisions on.
Each of these scenarios would require a different set of follow-ups for causal diagnosis which would be the first step towards rectification. The complexity increases as you go down the root cause reasons on this list with #3 being the most difficult to address. Managers are hired and paid for their judgement which they need to use in conjunction with data to ensure their teams and organizations are engaging in the right activities. A diagnosis of #3 would mean that an entire group of employees had been focused on a non-value-add activity that consumed valuable resources but didn’t help move the business forward. Now imagine if the manager had set the KPI for this initiative or business process to be based on a lagging metric (e.g. revenue achieved) instead of a leading metric. At the end of the period, the manager would have missed the target, would not have a clear diagnosis as to why the miss occurred, would not have clarity on which leading activities were pursued and to what degree of rigor they were pursued with and would at best have a highly subjective and speculative point of view on how they plan to turn the ship around in the next period that isn’t likely to instill a ton confidence in their superiors. The business review meeting would likely be a disaster. In a high-performing organization, this manager would likely be out of a job as well, and rightfully so.
What I’ve found is that in the modern workplace, every manager is likely to know their outcome KPIs inside and out (especially if their compensation is tied to it). The difference between an average and great manager is that an average manager will stop there and throw a bunch of darts at the wall to see what activities in a long laundry list might move the metric. A great manager will have a much more sophisticated and causal understanding of the highest leverage leading activities and their impact on lagging indicators and will manage their orgs to the best leading indicators with a high degree of confidence on the relationship between the two. This doesn’t mean that they aren’t constantly iterating on the inputs and fine-tuning the machine. What it means is that they approach initiatives and operational processes with the same sophistication and precision that a Formula One crew approaches a racecar when preparing it for a given race and during pitstops. That’s the common behavioral pattern I’ve observed in highly successful metrics-driven managers.
This brings us to the second most common mistake around KPIs which tends to be more insidious than the first as it often originates from a place of good intention. This mistake entails assigning a portfolio of contradictory KPIs to an initiative. There are many different definitions of strategy that are each valid when discussing the topic at varying levels of altitude. However, the common theme is the notion of scarcity, choice and tradeoffs. The essence of strategy and ultimately execution comes down to pursuing option B in a world where options A through F are viable choices. It’s a well-established fact that pursuing one option with complete focus, commitment, and team alignment results in outcomes that are better than trying to straddle multiple options with less than complete focus. For example, if you have resources for geographic expansion into 1 new region, it’s better to focus entirely on successfully executing that effort as opposed to trying to split resources and focus on two regions which will result in sub-part efforts, resourcing and outcomes on both. The same is true when you’re trying to decide what feature enhancements to prioritize on a product roadmap. Choice-as-the-essence of strategy has become a well-established and mainstream enough management principle to where most managers get it and adhere to it. Where I’ve observed managers trip up though is when they are translating strategy into initiatives and establishing success measures for a single initiative or business operation that are contradictory and involve inherent tradeoffs. This could entail a success measure for a launch that tries to optimize for both distribution and monetization (an inherent tradeoff), or a GTM strategy that tries to optimize for absolute growth and efficiency (an inherent tradeoff). There might be alignment at the objective or goal level on the importance of a discrete product effort or a partnership or a distribution approach (which adheres to the strategy = choice & tradeoffs principle), but it’s at the KPI level where the tradeoff and choice maxim gets consciously or subconsciously violated.
I believe that a core responsibility of managers at all levels is to absorb at least one level of ambiguity and abstract that into a degree of clarity for their teams. Nowhere is this more important than when trying to establish clear goalposts for success. Establishing contradictory metrics is like having multiple goalposts and switching between them based on what is important on any given day. Regardless of one’s best effort or intentions, there will always be situations that arise when those competing priorities are in conflict and one must choose how to navigate the tradeoff. If the rules for navigating the tradeoff are arbitrary, you can see how this would create tremendous confusion and churn amongst teams.
Now at this point, you might be thinking that most real-world strategic initiatives and business processes realistically need to have more than one objective. If you are launching a new product, it’s fair to have a desire to hit both customer count goals and monetization goals (which involve tradeoffs). Similarly, if you’re launching a new GTM strategy or channel, it’s reasonable to have objectives around both absolute growth and efficiency (again a tradeoff). Those are not unreasonable expectations to have. The key however is in how you approach, think about, and phrase the competing objectives. What I’ve concluded is that it is absolutely critical for any strategic initiative or business process to have one single “North Star” KPI and for all other competing metrics to be thought of as constraints instead of metrics to optimize.
As an OR/Systems engineer by training, I’ll borrow a concept from the field of mathematical optimization called linear programming to explain what I mean here. LP problems are essentially an effort to maximize or minimize an objective function given a set of constraints. For example, a farm owner may have an objective to maximize crop yield subject to some constraints in the amount of fertilizer and pesticide available. This is a classic LP problem. To solve for the optimal point, it is critical to think of crop yield as the primary KPI to be optimized and the fertilizer/pesticide as a constraint and not a metric to also be optimized. From a causal relationship standpoint, the fertilizer & pesticides are the inputs and farm yield is the output. If we revisit the common business scenarios above with this lens of delineation, we can think of one scenario in the product launch example as having a primary KPI of optimizing reach (# of logos) subject to a constraint of Average Deal Size (ADS) which will drive the monetization goal. The monetization goal will be met if the constraint is adhered to, however, it is not the primary KPI to be optimized and that distinction will determine the rules of engagement via which to approach the tradeoff when conflict between the two inevitably arises. Alternatively, it could be that the primary goal is to maximize monetization from the launch subject to a constraint around distribution / number of logos. This would warrant a different set of rules for navigating tradeoffs when the two are in conflict. When it’s clear that the target on the primary KPI will not be met by staying within the constraint conditions, it is the job of the manager to determine whether 1) The constraint should be mostly adhered to by reducing expectations of performance on the primary KPI, or if 2) The constraint should be relaxed somewhat to have a better chance at meeting the primary KPI. This tradeoff will be highly contextually dependent as you would expect. Note, neither of these moves absolve the manager of missing their commitment. Rather, they are a way for them to try and react to an unfortunate reality and salvage the situation in real time.
With this type of approach, it’s much easier to keep an objective score of success. In an ideal situation, the primary KPI target would be met without violating the constraint conditions. This situation would be considered an unqualified success. Alternatively, it could be that the primary KPI was met but it required exceeding the bounds set for the constraints (e.g. the target for new customer signups for a product launch was met but the ADS goal was not, or sales growth rate met the target but sales efficiency rates were not within the target thresholds). This is a partial success that warrants diagnosis on understanding what caused the miss on the constraint. Lastly, it could be that the primary KPI was not met and the constraint thresholds were violated as well. This is obviously the least ideal of all situations to be in and would constitute pretty unambiguous failure on the part of those involved.
I will make one additional point here about the notion of having a single primary KPI. This should be thought of as a point-in-time North-Star KPI and not a set-for-forever KPI. In fact, this KPI is almost guaranteed to change as the lifecycle of the business process, business initiative or business as a whole evolves. Here’s a real-world example that continues with the product launch theme. All of the major consumer internet services today (social media platforms, marketplaces, delivery services etc.) at their nascent stages were purely focused on user acquisition as their north star KPI. Monetization was a distant afterthought for most and hardly even met the threshold of constraint. Given Metcalfe’s law, the idea was to achieve ‘scale’ as quickly as possible before turning their focus to monetization. Once they hit scale, the focus, tactics, and importance of monetization changed pretty quite significantly at companies like Linkedin, Youtube, Uber and Doordash. In retrospect, this phased approach of having different north star KPIs at different lifecycle stages worked very effectively for these companies and they managed to easily outcompete rivals who had tried to maintain a dual conflicting focus on distribution and monetization before achieving scale.
In the next section, I will go over three discrete real-world scenarios at Alteryx where we pursued the wrong KPI for an important initiative due to a failure to understand the relationship between leading and lagging indicators, and where friction between conflicting KPIs created adverse behavior and outcomes and risked derailing our strategy. These experiences and lessons are what helped crystallize my thinking and perspective on this topic
AYX Case Study 1 – Partner Channel: Faulty understanding of the relationship between cause and effect resulting in the selection of the wrong KPI and missed revenue / ROI goals
As part of Alteryx’s transition to a heavier enterprise sales motion a few years ago, we decided to elevate the focus and investment in our partner / indirect channel and make that a strategic company imperative. The indirect business had been humming along for a while but growing less than the direct business and represented a smaller share of bookings than was typical at companies of our size. The program hadn’t really been optimized or re-designed in several years during which time it had grown in scale substantially and become a bit unwieldy. The partner types, tiers, rev-share thresholds, product packaging and engagement model all needed to be revisited and finetuned.
This is not meant to be a deep-dive on partner/channel strategy so I will skip over many of the details, but at the highest level, it is helpful to understand that for software companies, partnerships generally tend to fit into three archetypes and create value in one of two primary ways. The first archetype of partners tends to be value-added, distribution-centric resellers. These are partners who tend to have expertise in a specific domain, provide services which they can bundle your software with or have large master contracts with enterprise customers that can be leveraged to transact your product saving valuable time on contracting/legal cycles. The last of these is especially valuable for large customers where new vendor and MSA setup is a very involved process. They can vary from very niche boutiques with a handful of employees to national scale operators like SHI and Carahsoft. The second flavor of partners are other high tech / software companies. These are adjacent players in the ecosystem whose products have a complementary value prop. Partnerships with such peers often involve bespoke product integrations that make the products easier to use together and collaboration in the field amongst reps on specific account opportunities where the joint value prop is strongest. A good example of this type of partner for Alteryx was Tableau and Snowflake, whose products collectively comprised the ‘SALT’ stack. The third category of partners tend to be ‘System Integrators’ (SIs). These are mega-scale technology service providers like Accenture/Cognizant etc., the consulting practices of the big-4 and to some extent the big-3 strategy firms. The scale, reach and relationships of these firms with the upper echelons of management at the Global 2K make them a unique category of GTM partner that requires much more strategic engagement than traditional resellers. If activated correctly, they have the power to create tremendous leverage and create entire services practices around a software company’s product portfolio.
Partnerships can create value in one of two fundamental ways. The first is the following. By including a partner with unique domain expertise in an area that is relevant to your customers’ use-case for your product, makes your overall value prop as a software vendor stronger. The presence of a partner could result in a potentially larger deal upfront (vs. without them) and their role in ensuring the success of the deployment post-sale can drive future upsells and cross-sells which fuels the all-important net retention engine. They get a cut of the deal or sell additional services on top which makes it a win-win proposition. The second way that partners create value is by bringing you net-new deals that your sales team would otherwise would not have access to either from a different segment of customers or by leveraging unique relationships and opportunities within the existing base. This is a more direct benefit and is a massive source of leverage for software companies as it helps directly scale revenue without the need for incremental sales capacity. The best designed partner programs in software have activated a flywheel to where they benefit from both sources of value but particularly so from the latter. The early Oracle/Accenture, Databricks/Microsoft and Celonis/SAP relationships are great examples of such flywheels across different partner archetypes.
As we looked to scale up, increase investment in, and revamp our channel efforts, we knew that we would need newer and larger scale partners and would need to engage them differently than we had done before. We also believed that to get to that ultimate PIO leverage flywheel, we would need to “give in order to get”, which is to say, we would need to bring these partners into our current deals and give them a cut before we could expect them to bring us a plethora of net new opportunities. Our primary investment in the program therefore became 1) the revenue-share percentage that we gave these partners when bringing them into opportunities (though we made sure it didn’t impact the amount of commissions a seller received), and 2) the hiring of lots of partner managers who would liase with these partners and our sellers to ensure appropriate engagement on the right opportunities. We recruited several new large-scale partners and top leadership emphasized the importance of engaging partners in every opportunity and even created incentives and SPIFs for sellers to do so. We created two core KPIs to track these efforts: AIO (Alteryx or internally Initiated opportunities) and PIO (Partner Initiated Opportunity). The first of these represented deals where a partner was brought in and the latter were opportunities that the partner brought to us. The immediate north-star outcome KPI became AIOs (both count and $ value) which we tried to maximize across partner archetypes, particularly distribution-centric partners. I’m simplifying a bit, but the fundamental cause-effect assumption underpinning this strategy was a belief that increasing AIOs will cause PIOs to increase due to partner reciprocity.
The strategy was coherent on paper and we largely executed it as planned. Within a year, we had scaled AIOs to over 50% of deals and within two years to almost 70% of all new deals. The costs of doing so was not trivial as these partners often received up to double-digit percentages of deal value reducing Alteryx’s net ACV. The problem though was that the PIO KPI didn’t follow in the manner we had assumed and planned. The majority of new partners did not reciprocate being pulled into deals with bringing us more deals (though some did). Additionally, there wasn’t great evidence to suggest that AIO deals where partners were involved were significantly higher value to Alteryx than otherwise and the presence of other conflating variables such as Marketing and other GTM programs targeted at many of these same opportunities made is hard to attribute the relatively small deal-value lift to partner involvement. Upon further diagnosis, we were able to discover the more specific attributes and dynamics that DID cause partners to reciprocate our efforts of bringing them into deals with their own sourced deals but it was only applicable to a small minority of all the partners we had engaged. In retrospect, this was an example of a miss in understanding the cause-effect relationship between inputs and outputs in a nuanced manner and therefore assuming something that wasn’t true. Had we understood and identified the markers of what drove PIOs upfront, the initial north-star KPI we had identified for the program would not have been all AIOs but instead a subset of AIOs applicable only to a subset of partners and opportunities that fit those eligibility criteria. This would have resulted in better concentration and scale of business with the right set of partners and much higher ROI on the overall program than what the decision to pursue an ‘all AIO’ KPI yielded.
AYX Case Study 2 – Cloud launch: Trying to optimize two competing metrics resulting in suboptimal behaviors and outcomes for both customers and the company
A core strategic priority for Alteryx involved transitioning the platform from one that was deployed on desktop and on-premise or via self-managed-cloud to one that was a true multi-tenant managed service SaaS offering. The company’s efforts here involved some stops and starts over the years. Here, I’ll focus on one aspect of the most recent effort to build and launch ‘Alteryx Analytics Cloud Platform’ that was based on the revised R&D strategy resulting from the Trifacta acquisition and entailed a split-plane architecture.
Cloud was an important strategic imperative for the company as it represented the future and next-generation platform, yet it presented several strategic challenges for Alteryx which I discuss in depth in other posts (see articles on re-platforming strategies & monetization and pricing and packaging strategy). One of the central strategic questions we had to answer was how to define success for cloud. After much debate, the exec leadership team aligned around defining success as: “Achieving product-market-fit and go-to-market fit for cloud”. This was based on the logic that in order for the product to be considered successful, it would need to be valuable to customers and organizationally, we would have to effectively operationalize the operating model to commercialize it, particularly given the new monetization model underpinning the offering and the more complex sales cycle it entailed.
The more interesting and challenging exercise involved translating this strategic intent into KPIs and doing so intentionally across both leading and lagging indicators which is what would drive behavior. The top-level outcome KPIs we landed on fell into two categories in line with the strategic goals. The first of these was “Customer adoption and contentment” and entailed metrics such as platform deployment rate of sold SKUs, 1-month retention rate of first-time end users, NPS and SUS scores. The second category of metrics consisted of commercial outcomes, mainly bookings (ACV/ARR), renewal rates and net retention. While it’s not unreasonable to want both of these outcomes, there is the potential for conflict to exist between these two. In retrospect, the error we made was not defining one category and specific KPI as the north star metric.
From a GTM standpoint, the core teams did a lot of work to identify ICPs and target segments for the product. We also built an overlay field team comprised of dedicated cloud sellers and SEs to accelerate traction during the initial stage of the product’s lifecycle. As is customary, these teams were quota’d on the product-specific ACV and were meant to interface with the primary reps (who were still the primary account-level relationship owners) on account-specific opportunities. Despite all the work done on “push” based account targeting, as all experienced general managers know, there is a certain demand (“pull”) from customers, a reality that is difficult to ignore. You have to be extremely disciplined to say NO to a customer if they want a product added to their order form even if you know they aren’t the perfect fit for it. The Product teams (rightfully) viewed adoption as the north-star KPI and the GTM teams (who had product-based sales quotas) rightfully viewed bookings as the north-star KPI. The mistake we made was that by not specifying which of these two KPIs was higher up in the hierarchy at the org level, we failed to establish the rules of engagement for when conflict between the two would arise which was almost inevitable. Not surprisingly, we had several very large and strategic customers with a large willingness-to-pay that raised their hand and ask for the product to be added to their order forms given our marketing efforts. We knew at the time the product wasn’t ready for them (i.e. it may not have been available on their preferred cloud provider, in their specific region or may not have had a must-have feature necessary for their bespoke use-case), but because of the focus on bookings as a top-level outcome we were chasing and had incented the teams around, we ended up selling it to them anyway with the expectation that the roadmap would catch up in short order. In some cases, these customers didn’t even do the full diligence upfront to recognize the limitations and only realized the gaps after the deal had closed. The outcome of this dynamic was predictable in retrospect and not pretty. Though we hit several commercial KPI targets that year, the product didn’t have the deployment rates we wanted, it didn’t meet the NPS scores or develop the reputation we wanted amongst all early adopters (many of whom weren’t the target ICP) and our cloud renewal-rates for customers who didn’t sign multi-year contracts were not where we wanted them to be.
Though there were a lot of lessons to be learned from this overarching effort, in retrospect, all of the less-than-optimal outcomes were a function of a root cause reason which came down to a failure to establish a single north star KPI and adhere to it with strict discipline. Had we recognized and explicitly articulated the cause-effect relationship between early PMF and GTM fit (i.e. our two-part definition of success) more precisely, we would have recognized that commercial outcomes should be thought of and modeled as a much more direct function of product maturity and a sequential rather than parallel objective and KPI. Furthermore, had we exhibited better judgement about the state of the product’s maturity at the time of slated release, we would have likely lowered the commercial targets, rightsized product-specific GTM investments for the first year and put even more emphasis around the notion that adoption needed to be the primary focus, at least in the first year, after which the decision could be revisited. Pursuing bookings as a North Star outcome metric on par with adoption in the very early stages was suboptimal for the business in the long run as it incented the wrong behavior of focusing on distribution pre-maturely and selling to the wrong segments if they were willing to buy. Yes, this alternative “adoption-first” approach would have resulted in lower year-1 bookings number and as a public company, made our cloud traction message to the Street a bit harder. However, the long-term, multi-year impact of this approach would have been a lot better in terms of our ability to build a durable recurring revenue stream powered by a positive reputation-driven flywheel as was the case with the original platform instead of having to deal with the opposite of that.
AYX Case Study 3 – Customer Success & Services: How failure to explicitly identify the right North Star KPI resulted in execution that was inconsistent with strategy
One of the direct byproducts of the transition from license + maintenance to recurring subscription models in software over the last 25 years has been the emergence of the Customer Success function. In a license + maintenance model, once the customer signs the bottom line, most of the cash exchanges hands and deployment becomes the customer’s problem while the vendor has a lock-in on the smaller maintenance revenue stream. In a SaaS business though, the incentives are very different. Because the customer is renting software vs. buying it, they have a right to revisit their purchase decision every renewal event be that monthly, annually or over multi-year horizons (depending on the contract duration). The software vendor of course is highly incented to have the customer renew. Furthermore, because SaaS models typically scale up rev-capture over time as a function of some core value metric (seat per user, compute consumption etc.) vs. legacy models that don’t, successful initial deployment is much more important and critical not just for renewals, it’s also the best predictor of upsells and cross-sells which is the bedrock of the all-important Net Retention metric in SaaS. For these reasons, the SaaS industry has seen the mainstream emergence of a post-sales field function (commonly called Customer Success) whose job is to ensure that customers who purchase the software are successful with extracting value from it. At the most basic level, this entails deployment of the software but it also extends to ensuring that the specific needs and use-case of the customer are appropriately met ideally with a pleasant if not delightful experience. As the function has matured, there has been more sophistication and stratification applied to this idea and it’s common at large organizations to see multiple tiers of post-sales groups ranging from traditional success managers to solution engineers to resident consultants and professional service experts and so on. The lines between some pre and post-sales functions can get blurred at times.
While almost every SaaS org invests in post-sales resources to some degree even at the earliest of stages, a common pattern among high-growth SaaS businesses during the scale-up to moderately-scaled phase, is to outsource the more involved professional services associated with extracting value from their software to partners. The reason for this is simple. Services are a low-margin business that scales linearly whereas software is a high-gross-margin business that can scale exponentially. Given finite resources, a software vendor can expect better ROI on their investment by focusing on software and bringing in partners for the services piece. Additionally, investors across all stages value software revenue as an order of magnitude higher than services revenue. This creates a clear incentive to prioritize. When you hear a software vendor articulating an intent to “build an ecosystem around their platform”, a large part of that effort consists of getting value-added resellers, SIs and other service providers to prioritize service delivery for that vendor’s software offering thereby resulting in a positive demand flywheel for them.
That said, while delivering services is not the primary focus for software organizations, they do invest in some degree in services capabilities that can typically range anywhere from 5-20% of ARR once they cross a certain scale threshold to optimize the model before federating it. A few years ago, when Alteryx was approaching the half-billion ARR threshold, one of our takeaways during the strategic planning cycle was how the company under-indexed its peers in all of our services investments from traditional customer success to Pro-Services etc. This was interesting because the company back then had top-pixel Net Retention rates of 125%+ and license utilization and deployed workflows were the best leading indicator of account expansion. Since Customer Success was the most effective operational lever for improving utilization rates, this suggested a potential upside to growth if the unit economics of incremental investments there made sense. That insight, combined with the increasing levels of complexity of our product portfolio and its technical deployment needs suggested we’d likely have to scale up our post-sales and services investments.
As with any strategic initiative, it’s important to define success upfront. The core question confronting us was whether the services business was intended to be a direct revenue driver or an indirect revenue driver (i.e. by accelerating the software business). The answer to this question had enormous implications on how we thought about and measured this investment and impacted all subsequent downstream decisions which is why it was made at the executive level with the appropriate considerations. We reached executive alignment that the objective was to have it be an indirect revenue driver (i.e. help drive more software sales). Below is a visual that highlights the differences in operationalization of these two strategies
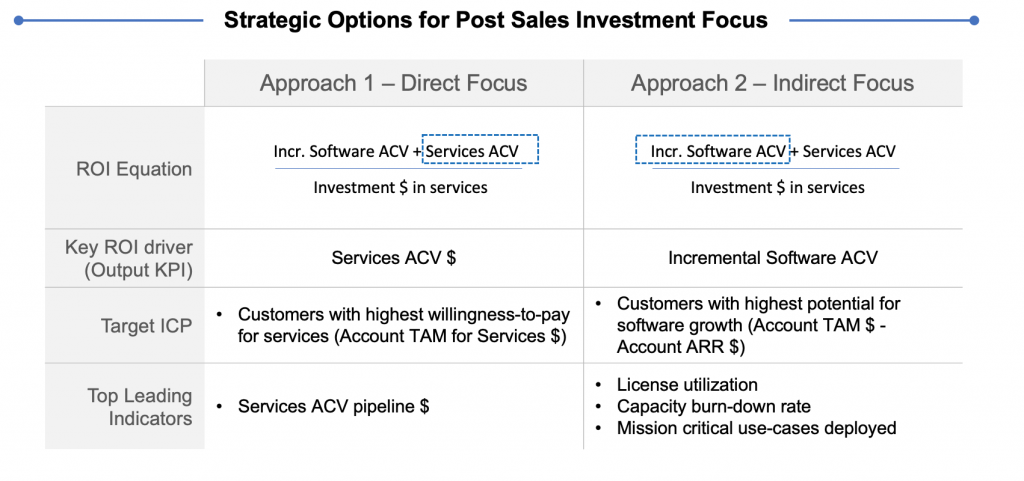
Though the ROI equation for total return on services investment remains the same in both scenarios, the component of the numerator that drives the equation is different. In the direct scenario, it’s the actual Services booking ACV $ that is the principal ROI driver. In the indirect scenario, it’s the incremental software ACV portion in the numerator which serves as the principal ROI driver. Each strategy has different implications on the corresponding ICP and set of leading indicators via which you operationalize it. By agreeing to an indirect priority, we were agreeing in essence to prioritizing all that approach #2 entailed.
When it came time to operationalize the effort, the question that surfaced was could we do both (i.e. monetize services AND drive indirect software growth)? This is always a tricky question and requires looking at the intersection of target ICPs under both scenarios in an intellectually honest manner. We undertook this exercise in 2021 and the reality of our business at the time was:
- We had a very low monetized recurring services penetration rate, even in mid-to-large accounts that would traditionally be willing to pay for some of this
- These accounts had plenty of untapped software TAM upside for upsell and cross-sell
- The macro was incredibly advantageous. Rates were low, capital was cheap, valuations were at an all-time high, profitability was the last thing on investors’ minds and most importantly, customers were willing to invest significantly in this category
We ended up concluding that yes, we could feasibly achieve both objectives and for the sake of simplicity, instrumented the program around direct success measures (which as stated above are a LOT easier to instrument, operationalize and objectively measure vs. indirect measures). This meant that Services ACV $ became the main output KPI and the Customer Success reps hired were incented and KPI’d around driving Services ACV pipeline and to a degree, overall account ARR. We did measure “excess” software net retention for this cohort as well (indirect benefit) but it wasn’t an operational metric. Now, granted there was a lot of low-hanging fruit to grab, but even so, the results that first year were incredibly impressive and we did indeed end up achieving both outcomes. We ~4x’d recurring services bookings (ACV) and the dollar-based Net Retention rate for the software portion of these Customer Success / Services accounts was ~20-25 pts higher than a similar group of accounts who didn’t receive this investment (the range being a function of attribution model selection).
Not surprisingly, when it came time for next year’s planning cycle, there was broad consensus on expanding the success/services investment further and sticking with the strategy and playbook that had worked. That’s precisely what we did instead of re-operationalizing everything to be based on indirect value (per the original intention). Three things however had (or would) change the following year which we didn’t recognize at the start of the cycle:
- Though still relatively low, our recurring services penetration rate had increased and much of the low-hanging fruit (obvious account candidates) had been picked
- The untapped software TAM upside in several accounts that still had a high willingness-to-pay for success services was much lower, especially since many had undergone large software upsells and didn’t have a high enough burn-down rate to consume it all in one annual cycle. Said differently, the number of accounts at the intersection of ICPs for the direct vs. indirect strategies was no longer very large making it unrealistic to assume we could optimize BOTH objectives with the same strategy
- The macro would do a 180 in the first half. Rates would increase, valuations would plummet, profitability would come back in vogue and customers would scale back on software spending
Not only had we continued the approach from the prior year, but the increased focus on overall business profitability resulting from the macro also had us leaning even harder on the direct monetization side of the equation. Somehow, direct P&L profitability for Services became a metric that started to become more front and center and we even went so far as to adjust seller comp plans to include a services quota component. By not appreciating those 3 key changes in context, we didn’t appreciate the distorted incentives that we were creating. The outcome was what you would expect. Sellers and CSMs focused on activities and accounts that would warrant higher direct services revenue. Not only did this result in a focus on less-than-ideal accounts from an indirect perspective but also caused Success Managers to go further up the spectrum of bespoke and non-scalable services that were lower margin. As a result, that year, we ended up increasing our services business by ~2.5x but the software net expansion of the cohort (which represented a minority of accounts but majority of the overall ARR) went from 20-25 pts above the equivalent non-services group to less than 5 incremental pts alongside a sharp deceleration of the overall business to half of its ARR growth from the prior year. This may seem like a not-so-bad outcome on the surface given the 2.5x growth of the services business on an ACV basis especially as it was right in line with the target set as a primary KPI. However, what if I told you that 1 pt in software ACV growth was equivalent to ~19 pts of services ACV growth for this group (given the delta in baseline) and that the difference in margins was ~25% vs. ~88% of revenue (another 3.5x). When measured on a contribution profit basis, we would essentially have needed the recurring services business to ~12x in size (vs. actual growth of 2.5x) to offset the entirety of the software ACV growth deceleration compared to the prior period. This no longer makes the 2.5x growth outcome look so good anymore despite the direct services bookings number being in line with the target.
The ultimate kicker in all of this was that the success and services teams (right up to the senior most executives) thought they had done a great job that year by meeting their target and felt they had finished the year on track to exceed their OKRs (2.5x service growth). This, despite the fact that the overall software ACV contribution from this group was nowhere near where it needed to be. So why the fundamental disconnect? The software growth contribution miss wasn’t top-of-mind for this success & services group and its top managers because despite the original strategic intent of using services as an indirect driver to enable software sales, by operationalizing it with a direct revenue objective we had completely divorced execution from the primary strategic intent of this effort.
That in a nutshell, is the consequence of selecting and operationalizing an imperfect KPI. Though it wasn’t the only reason, of the ~15 pts in overall ARR growth deceleration we saw that year, the majority of that was due in large part to a seemingly innocuous assumption that a continued focus on driving direct service bookings growth would yield the same indirect software growth benefits as the prior year. We had lost sight of both the cause-effect relationship, the activity-to-outcome relationship and hadn’t fully appreciated the evolved account-level dynamics that invalidated the original assumptions. A deteriorating macro of course was the accerlerant on top of all this which greatly amplified the effects.
The fundamental lesson this experience reinforced was the following. Initiatives that are intended to drive indirect benefits need to be instrumented and operationalized with more sophisticated (and often more complex) KPIs that capture their indirect benefit through some type of reasonably objective attribution model. Despite a strategic intent among senior leaders to pursue these as indirect benefit efforts, it’s very easy and tempting for managers to operationalize success measures for such efforts using traditional direct revenue and cost-centric business case KPIs (as it’s much easier to do so both mechanically and when justifying the outlay to the CFO). It’s also very tempting for managers to convince themselves that they can avoid tradeoffs and achieve multiple objectives (i.e. achieve both the direct and indirect benefits) despite evidence suggesting that the ability to do so is the exception & not the norm. The urge to pursue both benefits instead of the primary one should be resisted similar to how the urge to straddle any strategic choice should be resisted. At best, you will dilute the intended impact of your effort. In the worst case, you will destroy value by distorting incentives and creating behaviors that don’t align with the original strategic intent underpinning the effort.
—
In summary, I’ll conclude with the following. There’s been no shortage of ink spilled on the topic of ‘Strategy’ in the business press. Furthermore, consensus opinions on the importance of execution and operational rigor have only converged and trended up with a rightful recognition that this is ultimately what separates winners from losers. In my view, there has been much less discussion around how to correctly translate strategic intent into appropriate performance indicators despite this being where the rubber actually meets the road and the extremely high leverage nature of the exercise. Having attended an okayish MBA program (albeit a while ago), I can tell you with certainty that the program had no shortage of focus on both the strategy and execution themes. I don’t however, recall much about the selection of optimal performance measures. My hope is that these examples based on first-hand experiences from the trenches, can convince managers and business operators of the criticality of selecting the right KPIs and can help them navigate the pitfalls of translating strategic intent into desired action in what are intrinsically messy and unpredictable human-centric systems.