(~25 minute read)
—
In early 2019, my role at Alteryx entailed overseeing a small, yet high-caliber team focused on Corporate Strategic Planning, Corp Dev and BizOps. I was reporting to the Chief Strategy Officer at the time. Upon her departure, I spent about 6 months reporting to the founder and CEO. It was an intimidating yet exhilarating experience as we completed two key acquisitions into important adjacent areas, launched a major new product and grew the top-line 65% on a baseline of ~$180M in ARR while we 3x’d our market cap to almost $10 billion. I fully expected this reporting structure to be a temporary arrangement so I was surprised when the CEO at the end of the year said to me “Junaid, I want you to be a permanent member of my leadership team as a VP and my Chief of staff”. I was pretty taken aback and quite skeptical. This wasn’t the path I had in mind, I wasn’t at all familiar with this role (outside of politics) and I had dozens of questions swirling in my head that I wanted to ask. What does this title mean? What does the role entail? What’s the actual remit? Is this open-ended or time-bound? What happens to my team? How would comp and RSUs get impacted? What the heck would I do after this? You get the picture. After taking a few seconds to compose myself, I decided to respond with the highest information leverage question I could think of in that moment, which was, “OK, if there is just one thing you want the person in this role to achieve, what would that be?” Without hesitation, Dean responded, “Advising me on high stakes decision making to ensure that we make the right ones in a timely manner when it really matters. Complexity in the business has increased dramatically and will only intensify further on our path to $1B+ and I need someone deeply analytical and with good judgment, who can see the big picture and whom I can trust to help me and the executive leadership team navigate it. You are unique in possessing all of these traits”. While flattered, I still had tons of follow-up questions, concerns, and hesitations. Yet despite all of them, in that moment, I was essentially sold on taking on the role. By the way, I learned later that this is not what most “Chief of Staffs to CEOs” in business do so the title ended up being a misnomer and not quite reflective of the role, but that is a topic for another post. I also didn’t realize it at the time, but I had just signed up for a cockpit seat on a dizzying 5-year public company journey from ~$250M to $1B in ARR with visibility to and influence on every consequential decision along the way accompanied by tons of drama which included a founder/CEO succession, a reset of the entire executive team, a platform transition, a GTM transformation and ultimately, a change of control! 😳
So why was I excited in that moment?
Decision-making is a topic I’ve long been fascinated with which has led me to consume much of the literature on the subject from the classic works of greats like Kahneman and Tversky to the more contemporary frameworks offered by high-stakes decision practitioners like Annie Duke. In a nutshell, it’s because I prescribe to the Bezos philosophy that “at the end of the day, where you end up in life is, for the most part, a function of your portfolio of decisions”. This rings true for me in both the personal and professional realms. In many ways, my decade of prior experience in management consulting could be summed up as helping senior execs make the right decisions on consequential matters, so this is an area I had some expertise in and had developed some mental models around. That said, despite the fancy college and MBA degrees I had pursued, the prestigious brands of the firms I had worked at and the blue-chip portfolio of clients I had taken pride in advising over the years, I didn’t feel as though I truly had ‘skin in the game’ on consequential decisions and real accountability & exposure to the outcomes. I figured this opportunity would 1) Let me observe up close and learn from the decision-making approach of an entrepreneur who had taken an idea from the back of a napkin to a ~$10B market cap company, 2) Be in a position of driving value-added influence on the truly tough decisions and have real exposure to the outcomes – the latter being something you don’t ever have as an external advisor regardless of how lucrative that path may be.
The fundamental job of a senior executive in business is to make important decisions that result in the outcomes desired by the company. The more senior the role of the exec, the more leverage their actions have and the more consequential their decisions are going to be. Getting it right drives big upside while getting it wrong often results in significant value destruction. When we say that someone “has the right experience for a job”, we are implicitly saying that their experience has enabled them to develop the judgment needed to make the right decisions in a particular area. For a CEO, that area is the entirety of the business. In my role, I’ve had the opportunity to work closely with 2 very different CEOs (an entrepreneur/founder and a professional manager), two different executive leadership teams (as the entire original team transitioned with the first CEO), and a group of 30+ SVPs and VPs on critical decisions that touched every function and corner of this business.
In hindsight, during this period from $160M to $1B in ARR, we got several decisions right and several decisions extremely wrong. In fact, this blog contains 15 business lessons structured as case studies that go deep in the cause-effect relationship of specific decisions on outcomes across many different facets of the business (platform strategy, hiring, GTM org structuring, monetization, M&A etc.). Different domain spaces obviously require different decision making considerations, however, what I challenged myself to do was to see if there was a generalizable framework that I could synthesize as a lesson from the process I observed the best decision makers undertake across domains. This could serve as a guide to maximizing the likelihood of making the right ones. I also tried to reflect on why we missed on the big decisions we got wrong. The model below is where I landed on this effort. Note, I’m not aware of anyone who had explicitly codified and formally used this model for decisioning. Rather, it’s derived implicitly from the approach that I observed effective decision makers undertake when facing important decisions and situations I was involved in myself. Also, please note, this model doesn’t get into governance around decision ownership and accountability. There are plenty of good models for that and I address that separately in another post (particularly the issue of aligning accountability with decision rights). Instead, this model is focused on how to approach the actual process of making high-stakes decisions.
The rest of this post systematically decomposes this model with relevant examples. I then share a perspective on what types of evidence to lean harder on and which ones to avoid for different categories of decisions.
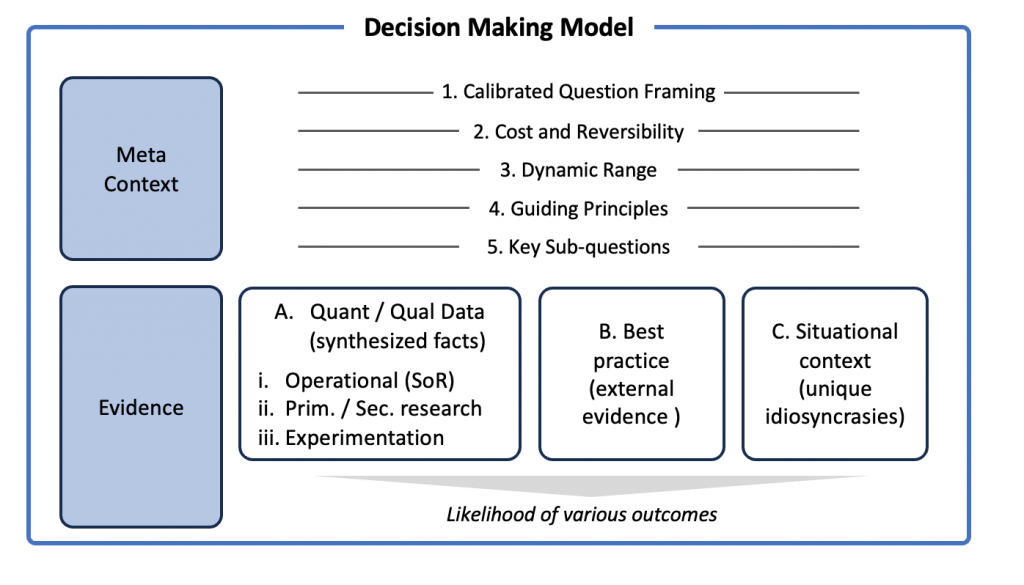
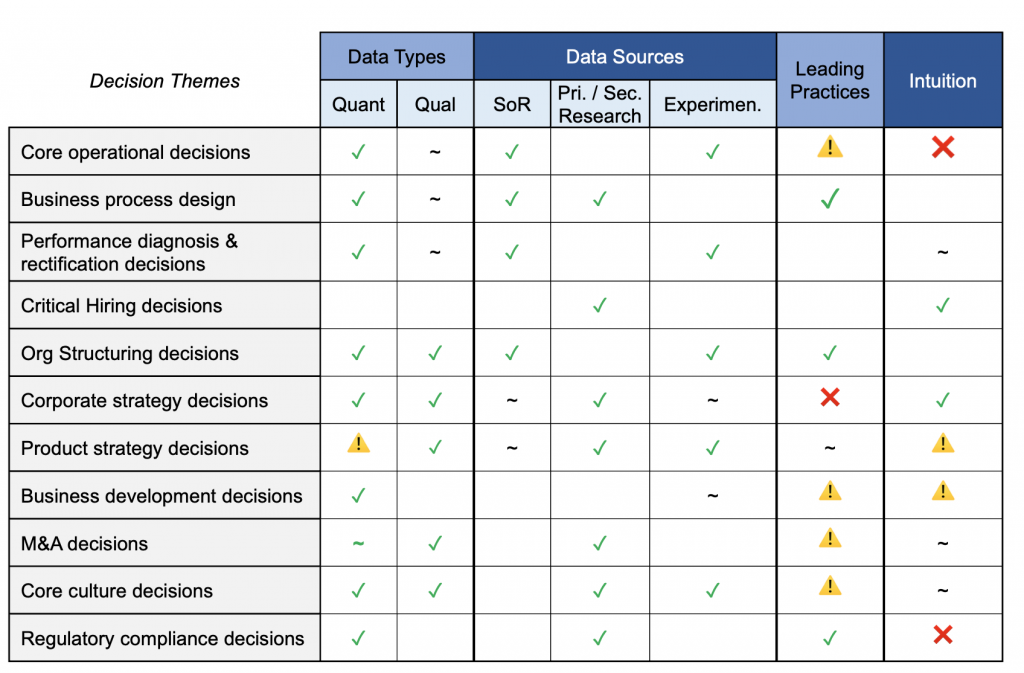
I tend to think of important decisions as having two principal components. The first component is meta context which entails a set of meta decision considerations and the second is evidence which consists of facts and realities in favor of or against pursuing different decision scenarios. One of the things that immediately jumps out from looking at this visual is that so much of what constitutes effective decision making is ‘meta context’, i.e. perspective on the decision to be made. This is somewhat contrary to the overwhelming focus on evidence-based or data-driven decision making which tends to dominate discussions on decision making. Don’t get me wrong, evidence is incredibly important, however, it is only valuable within a given context.
Part 1 – Meta Context
Starting with the context dimensions, numerous studies have shown that decisions are significantly influenced by the way they are structured. Since important business decisions mostly take on the form of questions (i.e. “should we do X”), it’s imperative that the question be framed correctly to ensure that you are answering the right one and it’s free from bias. This is where widening the aperture really helps and why senior leaders are expected to tackle the hardest decisions. It’s because they have the broadest aperture with which to frame and contextualize the real question that is often underneath the initially posed question. Here’s a more concrete example. A few years ago, we were debating whether to pursue acquiring a company primarily as an acquihire. As leader of Corp-dev at the time, as I ran through the usual deal considerations in the materials for the committee, I was interrupted by the CEO who asked me what decision were we really making, to which I responded, ‘it was whether or not we should acquire X’. At that point, he helped clarify that the real decision we needed to be making was ‘how do we quickly get a critical mass of engineering talent with expertise in domain X up and running productively…. ideally in region Y’. Reframing the question this way provided a completely different perspective as it contextualized the puts and takes and made clear which risks were relevant and which were not and could be ignored. Figuring out the right question to be asking is paradoxically much harder than figuring out the answer to it with the returns of this exercise being extremely high leverage. Reframing the question is also often the most effective path forward in situations where there is gridlock among decision makers. The best decision makers are experts in decision framing and do so by ensuring the right question is being asked before engaging in debate.
Cost and reversibility is another import meta decision consideration. Perhaps the most well-known advocate of this dimension is Jeff Bezos with his famous type-1 vs. type-2 decision model. Simply put, type 1 decisions are those that are irreversible ‘one-way door’ decisions that carry a high cost and are tough to undo if you get them wrong. Costs here should be measured in both $ and time with opportunity cost often being a bigger factor than explicit capital outlays. These decisions warrant thoughtful consideration and debate (using more of what Kahneman would call the ‘System 2’ part of our cognitive faculties) and a higher threshold of evidence and confidence in likely outcome to proceed ahead with. Type-2 decisions conversely are those that are more easily reversible without exorbitant costs. It is acceptable to prioritize speed over high degrees of certainty in type-2 decisions because the benefits of moving quickly outweighs the cost of recalibrating later on if you get it wrong. In my observations, great decision makers know how to delineate between the two (which isn’t always clear cut given hidden opportunity costs), and delegate type-2 decisions to their teams to empower them to move quickly and help them build their own judgment muscle. What they ensure in these situations though is that such decisions are correctly framed and intervene appropriately when they are not. The types of decisions they tend to focus on themselves are of the ‘type 1’ variety. From my observations, type 1 decisions can encompass a pretty wide swath of themes from the more obvious (e.g. core product architecture, business model adjustments, disruptive M&A and recruiting senior roles) to slightly less obvious ones (e.g. establishing core cultural tenets, ensuring compliance with esoteric rev-rec rules and motivating and inspiring employees during periods of low morale).
Here’s a concrete example of this phenomenon during our journey. We crossed the $0.5B ARR threshold in early 2021 and at the time we had a negligible amount of services revenue, opting instead to let our partners manage the ProServ around our software. At this stage, it became clear that we needed an in-house services capability for our largest customers and building one was a strategic imperative for the following year. There was a ton of decisions to be made and work that needed to be done around architecting our services offering, figuring out how to package and price the services and ultimately operationalizing them. While important, these were mostly type-2 decisions. The type-1 decision that needed to be made was whether the services business was intended to be a direct revenue driver, a loss (or breakeven/poor margin) leader, or an indirect revenue driver (enabling the software business). The answer to this question had an enormous impact on how we thought about and measured this investment and all subsequent downstream decisions which is why it was made at the executive level with the appropriate considerations.
As a photography enthusiast, the next element of this decision model for me was inspired by a concept in photography. ‘Dynamic Range’ in photography represents the ratio between the darkest and lightest pixels in the image. The higher this is, the harder it is to accurately process the image and the more sensor surface area and power is needed. For business decisions, the concept refers to the difference in magnitude between the 80th percentile outcome and the 20th percentile outcome of the decision and is independent of the cost and reversibility aspects of the decision (though it can be correlated to those). The higher the dynamic range (the variability between the two ends of the outcomes), the more focus and deliberation is needed to weigh the evidence to get it right. An aspect of business where this concept is particularly relevant and helpful is during hiring senior or critical roles. Let’s say that as an exec, you are trying to assemble a cross-functional team to tackle an important and strategic AI product initiative. Furthermore, you know that amongst several key roles, you will need competent and highly capable engineering and privacy counsel leads to drive this effort to success given the emerging tech and ambiguous legal landscape this domain entails. Now, the reality is while both roles are important, and might even have similarly high price tags, the dynamic range of outcomes for the engineering lead role is likely to be an order of magnitude higher than the privacy counsel role. That doesn’t mean you don’t need an effective privacy counsel, you certainly do. What it means is that the amount of effort one should spend recruiting, vetting and overseeing the engineering leader (across your portfolio of hiring decisions) should be an order of magnitude higher given the greater variability in impact on the outcome of having a great vs. average person in that position relative to others roles. More broadly, I have observed that it’s not uncommon for managers to mistake type-1 decisions as type-2 decisions because they haven’t fully comprehended the opportunity cost and dynamic range of the decision which elevates it to be a lot more consequential than it appears on the surface.
The next contextually relevant meta element of decision making are guiding principles. These are critical because they make explicit the bigger picture objectives underpinning the decision, the common rules by which a diverse group will decide, and how to deal with tradeoffs. When approached correctly, effective guiding principles dramatically simplify the process of going from the ‘evidence’ gathering phase of a decision to a relatively consensus driven resulting action. Conversely, the lack of coherent guiding principles are often a leading cause of misalignment amongst decision makers and almost always result in contentious decision making processes. Here is a concrete examples of guiding principles in action. When Covid-19 first hit in April 2020, Alteryx’s business saw a sharp change in customer buying behavior and an expected decline in revenue growth. It became clear at the mid-year point that we would have to re-do our annual operating plan, reduce seller capacity and reset quotas for the field comprised of ~330 sellers at the time. This was not an easy exercise and we had three weeks to complete it without risking deterioration in unit economics, falling behind in Q3 or starting to see good sellers defect. Though Finance and Sales/Rev Ops would typically be the natural “owners” of this exercise, I was asked by the CEO to drive and oversee this initiative. His reasoning was simple. Sales would naturally be biased towards seller wellbeing in a situation like this while Finance would have a natural tilt towards the company’s financial wellbeing. He trusted I would bring a degree of objectivity to both sides of this exercise without over-indexing on one or the other. This was a difficult exercise given the exec personalities involved and put me in a tough spot. There were an infinite number of theoretical design permutations we could pursue for the remodel and massive uncertainty in the 2H outlook compounded the complexity. I asked him for counsel on how to approach this and he advised aligning on guiding principles with key stakeholders first. So, instead of jumping in the weeds of model design from the get-go, I decided to really hone in on the guiding principles and align with each key stakeholder (particularly CFO) on those well before we got to the stage of discussing different scenarios. We agreed upfront to several guiding principles, a subset of which included: 1) The three key leading indicators we would use to monitor the health of the business and how to translate those into confidence levels on top-line, 2) That rep level fairness was critical (i.e. sellers in NYC or those with territories heavy on hospitality/energy logos had to be treated differently than those that had telco or cybersecurity heavy bags), 3) That we would measure territory risk using a risk based model derived from a public market index in different geos (S&P500, FTSE100, Nikkei) and that we would vary overage buffers across segments based on magnitude of demand uncertainty vs. our prior policy of having standardized overage percentage at each layer of the sales managerial hierarchy, 4) We would aim to maintain our commercial unit economics (CaC and S&M efficiency) and target the same percent of sellers achieving quota prioritizing that over keeping a greater number of existing reps at the company. This upfront guiding principles alignment exercise dramatically simplified the permutations required during the modeling exercise and helped immensely with driving alignment on the final revised plan we landed on for the year. Though engaging in guiding principle discussions can feel like a luxury and a somewhat conceptual exercise especially when time constrained, it should be thought of as akin to sharpening the axe before starting to hack away at the tree with a blunt instrument.
The last important element of the model within the context pillar entails identifying ‘key sub-questions’. Real world business decisions are incredibly complex and multifaceted. While they can be of the yes/no variety and sound simple on the surface, arriving at the right answer to them requires weighing a multitude of considerations. Put simply, key sub-questions are the right underlying ‘high-information leverage’ questions which are more directly answerable and can collectively inform the overarching decision in a practical and actionable manner. Collectively, the sub-questions exhaustively capture all the important sub-components of the decision which based on the evidence (data) can help one make the overarching decision. This concept of logical decomposition (also sometimes referred to as the Minto pyramid) was pioneered by Barbara Minto in her book “The pyramid principle” and is one of the most foundational skills all top-tier Management Consulting firms instill in their incoming recruits to help them more effectively navigate complex problem solving in ambiguous environments.
Here’s an example of this element in action on a highly consequential ‘type 1’ decision we faced. As part of transitioning Alteryx’s platform to the cloud, we challenged ourselves to reimagine and re-architect not just the core product set but also the business model underpinning it. This meant approaching pricing and packaging with a clean sheet of paper with a very broad aperture, something that is not easy to do when you have a $1 billion ARR installed base of customers on the existing model. Many of you are likely aware of the big emerging trend of consumption based pricing over the last 5-8 years in the SaaS world (as opposed to the traditional subscription-based model). Though it was pioneered by infrastructure and API centric technology orgs, the consumption model in 2021 was starting to gain some traction with companies at the intersection of infra and application software and had demonstrated very favorable unit economics particularly on the critical net retention KPI. One of the questions the board and the CEO asked was ‘should we transition to a consumption based pricing model for our cloud offering? (vs. continuing down the subscription pricing path)’. This is a classic example of a broad and complex ‘type 1’ decision question that needs to be decomposed into key sub-questions before it can be coherently answered and actioned. This article is not meant to be a pricing-and-packaging deep-dive so I won’t go into all the sausage making here (see other ‘monetization lessons’ post for that). Broadly speaking, by decomposing the overarching question into customer preferences, the core revenue equation, the breakeven threshold, before and after customer ARR distribution and benchmarking the breakeven threshold rates against peers, we had an approach by which we could gather and apply the right quantitative and qualitative evidence to determine the answer to the question. The final outcome of the exercise is that we concluded that a pure consumption based pricing model was not a viable one for the Alteryx cloud platform, however, it made sense to include a consumption vector as part of a hybrid model to drive monetization upside. More broadly, what I observed is that the best decision makers who are experts in a functional or vertical domain space have an uncanny ability to immediately hone-in on the most critical sub-questions to ask to help inform a complex decision which their high performing teams can then help them answer with the appropriate quantitative and qualitative evidence.
Part 2 – Evidence
Having established rigor around the real decision you are trying to make, the objectives underlying it, it’s relative criticality, and how you will go about making it (meta context), bring us to the second and arguably more fun evidence pillar. A high-stakes business decision is at its core a bet on achieving one out of several possible futures on some consequential issue. Because it is a future outcome, there is by definition, always some degree of uncertainty involved. The spectrum of outcomes – even when they feel inevitable – are probabilistic, not deterministic. The overarching purpose of the evidence pillar is to inform a decision maker of the underlying facts and help them establish the likelihood of the different outcomes that could transpire in response to the different decision scenarios that are under consideration. The more rigorous your understanding of the relevant facts is, the greater is your chance of arriving at the correct conclusion ex-ante of what outcome is most likely, along with a thoughtful measure of the spread of uncertainty around it. This is not an easy task and is a skill that needs to be developed and carefully honed. Effective business execs tend to have a bias for action and their successful track records often give them the (sometimes misplaced) confidence to rely on their intuition when making judgements. It’s a lot easier, faster and lower effort for them to proceed based on intuition alone. The best leaders though, avoid giving in to this temptation when it really matters.
One of my favorite quotes on evidence based decision making comes from the physicist William Pollard. He famously said, “Information is a source of learning. But unless it is organized, processed, and available to the right people in a format for decision making, it is a burden, not a benefit”. It demonstrates succinctly why despite the broad consensus on the benefits of data-driven decision making and hundreds of billions of dollars spent by Global 2000 orgs on data and analytic infrastructure, the elusive practice of evidence-based driven decision making is still very much the exception versus the norm. So how does one go about leveraging evidence for decisioning?
It begins with recognizing that there are several flavors of evidence that each serve a slightly different purpose. The first of these is ‘data’ which is a collection of facts and statistics. Data can be quantitative or qualitative in nature. Quantitative data is numeric, easily measurable and often structured giving it a semblance of objectivity. It’s why quantitative data is the bedrock of all scientific research and inquiry. Qualitative data on the other hand is non-numeric and mostly unstructured (made up of words, descriptions etc.) giving it a degree of subjectivity. It can often get conflated with “anecdote” however it’s important to recognize that these are not the same thing (though there is often a slippery slope between the two). Hearing from a prospect that they didn’t purchase a product because it was too expensive is an anecdote. It’s dangerous to generalize this feedback into a broad ‘truth’ upon which to base a decision. Alternatively, systematically interviewing 50 customers across different segments on what drove their purchasing decisions is a rich qualitative dataset. Learning that 35 of the 50 hit on a common theme of underlying purchase driver makes it much more likely that you have surfaced an insight that represents a foundational truth that is incredibly helpful for decision making purposes. Quantitative data is typically helpful in establishing objectivity around a ‘what’ or ‘how much’ themed question, while qualitative data is helpful in providing color and context around the ‘why’. Despite the traditional view of quantitative data being ‘superior’ to qualitative data, I have increasingly come to appreciate the importance of the latter after seeing how great decision makers utilize it in conjunction with the former. Complex decisions almost always require a combination of both.
In addition to these two data types, it’s important to recognize that data can come from different sources. I tend to think of data as generated from three primary sources. The first is internal data. This is data generated by the company most often through its various systems of record (CRMs, ERPs, marketing systems, etc.) and can be quantitative (e.g. transaction quantities and prices) or qualitative (e.g. notes from open ended CRM fields). This type of data represents history and is fundamentally an “inside-out” view of reality. Given the complexity and multi-faceted nature of real world business, it is generally not possible to infer causality from this data. The second type of data is research based data. This is data that is generated by primary or secondary research on a subject and represents an “outside-in” view of the world. The third type of data is experimentation data. This is narrow-scope, highly specific and curated data that is generated via bespoke experiments in an attempt to to answer a specific question. It is the gold standard when trying to infer causality between two variables and why it’s used extensively in domains such as Pharma by regulatory bodies to approach high stakes decisions like whether to approve a new drug. Low-cost experimentation data is one of the richest and highest quality signals of evidence that the best decision makers rely on whenever possible. In fact, the importance of experimentation is one of the bedrock elements of organizational culture when it comes to decision making in modern, high-performing digital native organizations. If it’s possible to collect high quality statistically predictive experiment data within a week to inform a decision, managers at such companies often have an expectation that it will be done and made available at an executive decision review to underpin a recommendation.
Each of these data sources have a different and distinct role to play in high-stakes decision enablement detailed in the next section.
The second major dimension of evidence based decision making is “leading practice”. While this has become a grossly overused business buzzword (particularly by consultants), the underlying logic behind the concept remains relevant. All business categories have a handful of companies with great track records that lead their respective categories and that often tend to approach core business activities differently. Understanding and potentially replicating the relevant behaviors underpinning their strengths that are relevant to another company can be helpful. For example, Amazon’s track record of new product and market entry is enviable. Apple’s prowess in UX is unparalleled. Microsoft’s track record of using bundling to beat the competition is legendary. Taking cues from Amazon around decisions involving how to structure product development or from Apple on decisions involving UX design or from Microsoft on how to approach enterprise licensing may not be a bad idea. Additionally, when it comes to high growth/ scale-up phase organizations, it’s common to find certain playbooks within different sectors that are generally accepted collections of ‘best practices’. These playbooks are often based on the trailblazing efforts of an industry pioneer which are then further codified by fast followers and other aspiring and motivated industry participants. As an example, in SaaS, the modern high-growth GTM playbook encompassing everything from sales org structuring, new logo acquisition engine design, international market entry, event strategy, customer success roles, community and marketplace strategy and much more were almost all pioneered by Salesforce. Similarly, the modern Product Led Growth (PLG) playbook was pioneered by Dropbox and perfected by Slack. These playbook themes include important (often Type 1) decisions every SaaS company will have to make. Referencing such playbooks as an external data point can be a valuable decision aid. It’s important to note however that such exercises need to be approached with caution as the business graveyard is littered with companies who had key execs that that tried to copy-paste the playbook they learned from BigCo at SmallCo without taking into account the appropriate context. We certainty suffered some significant failures at Alteryx as well due to this phenomena.
Once the right sub-questions underpinning a decision have been identified, the hardest part of evidence-based decision making includes determining:
- Whether data can even be used to help answer (or inform) the question
- If so, identifying what kind of data should be prioritized
- How should one synthesize the key takeaways and draw the right conclusion from it (the so-what) to inform the overarching decision
While the difference between the involvement of a great vs. average analyst has an immense impact on the effectiveness of this exercise (and is a big reason why great leaders value great advisors), the ultimate responsibility for this exercise lies with the principal decision maker and can’t be delegated. When we say that someone has great ‘judgment’ around decision making, we are essentially saying that they know how to navigate these three steps and overlay it with situational context and their intuition that’s been built on a broad base of knowledge and experience. This is displayed visually below.
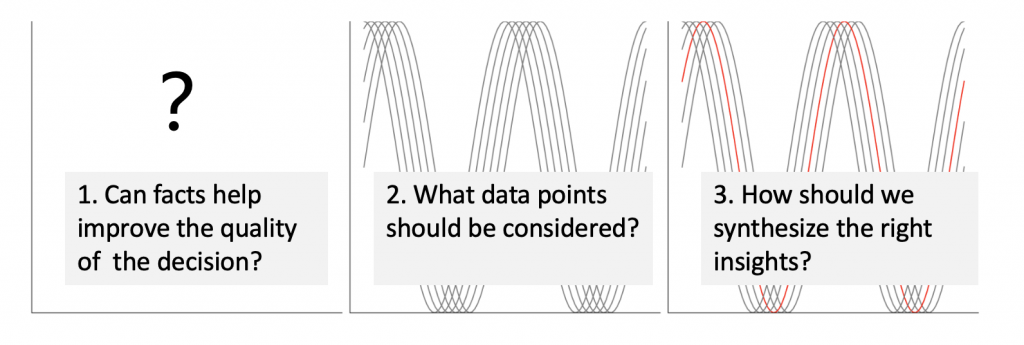
So how does one go about improving their business judgment? It requires practice and unfortunately, I’m not aware of any shortcuts. Good judgment is a byproduct of relevant experiences and learnings from both successes and failures with intentional reflection afterward. The good news is that there are heuristics that can help provide guidance on which types of evidence to lean harder on for different types of high-stakes decisions. The matrix below highlights the types and sources of evidence that should be prioritized and avoided for different themes of high-stakes decisions. ✓ indicate the primary types and sources of evidence that should be considered for each category of decisions, ~ represents useful secondary sources, ❌ represents sources that should be avoided and ⚠️ represents potentially useful but also hazardous sources of evidence that should be approached with caution. Collectively, these can help provide situational context to managers for different types of type-1 decisions
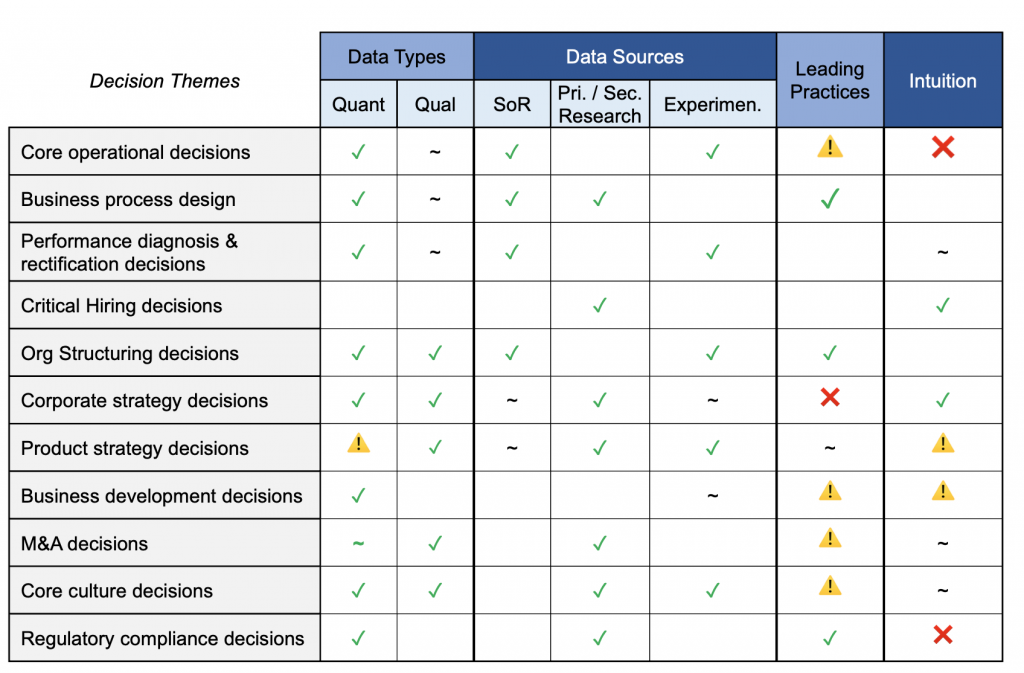
Core operational decisions tend to be the most common types of decisions that come up in a business context. Most of these are not of the high-stakes variety but some do rise to that level. These can represent budget allocation, capacity planning, offshoring , key vendor selection or workforce management decisions. Core operational decisions are really well suited to quantitative, inside-out data and tend to entail relatively lower levels of ambiguity. Business cases, risk assessments, demand forecasts etc. are all well-defined quantitative approaches to enable these decisions as are experiments. In general, it’s best to avoid relying on intuition for these in lieu of data. Leading practices also tend to be less helpful for such decisions as the inside-out context generally significantly overshadows the outside-in perspective.
Business process design decisions are generally not considered the sexiest type of type-1 decisions but are incredibly high leverage especially for mission-critical processes comprising an organizations core-competencies. For a SaaS organizations, this can entail the product development / new product introduction process or a demand generation / discovery-to-quote process. Inside-out quantitative data from systems of record can provide great perspective on current state strengths and gaps here but is almost always going to lag best-in-class and have a narrower aperture. Outside-in best practices are a really rich and valuable source of evidence for such decisions. If you want to maximize the likelihood of building and releasing successful products, it’s hard to go wrong by copying Amazon’s ‘working backwards’ framework and the associated PR/FAQ approach. Similarly, if an organizations wants to design an M&A integration business process, adopting Cisco’s playbook is likely to be better than recreating the wheel yourself. You probably won’t need the same level of breadth and overhead, but regardless of what stage of M&A you are considering, it’s likely going to be a subset of Cisco’s acquisition playbook given their depth of experience and almost scientific level of rigor on how to approach integration. Org structuring decisions are another category of decisions where leading practices can be helpful. If you’re scaling up a GTM org for a mid-ACV largely outbound SaaS motion with a short to medium sales-cycle, the average quotas, AE to SE ratios, BDR pipe-gen expectations, MQL to SQL conversion, and CSM coverage ratios will all be within certain benchmarks. Contextualization is still important and the benchmarks are ranges vs point estimates. However, if you find yourself outside these bounds, you should really drill in on why that is. The chances that you might be sub-optimized are higher than the opposite scenario.
Critical external hire decisions are a unique type of high-stakes decision. By definition, there is no internal data and experimentation isn’t really feasible so you really only have your interactions (qualitative evidence), reference checks (research) and ultimately your intuition to go by. Of the various types of high-stakes decisions, this is one that is hardest to teach and is heavily dependent on intuition and personal judgement. The best leaders and senior managers develop their own unique markers for identifying high potential talent in their respective domains. The below average leaders in my experience, tend to excessively over-index on the ‘BigCo’ brands they see on resumes which at best is an average predictor of transferrable success and often results in critical mis-hires which are one of the most expensive types of mistakes an organization can make. As an aside, I’ve observed that the hit rate with critical, high-dynamic-range early hires is the best leading indicator of how much success an executive will have at a given organization more so than how capable they are individually. The second best leading indicator is how quickly they get rid of an underperforming manager once it’s clear that they’ve made a mishire.
Corporate Strategy decisions are probably the highest stakes, most ambiguous and the hardest-to-get-right category of decisions. They are the epitome of Type 1, ‘high dynamic range’ decisions. These decisions often entail questions such as where to play, how to win, how to evolve the business model, how hard to chase growth, what adjacencies to pursue as second acts and whether to execute these via build-buy-partner etc. They entail incredibly broad and complex questions and therefore require the broadest aperture views which is why they fall to CEOs and the most senior executives. There is no perfect data source for these decisions and the only source of evidence to avoid entirely here is ‘leading practice’. If your organization is trying to compete in the CRM or ITSM SaaS space, the last thing you want to do is emulate Salesforce or ServiceNow’s corporate strategy as your survival will be premised on how much you can differentiate against these leaders. Internal evidence can be helpful to establish some truths though outliers matter more than averages here and outside-in data (both quantitative but especially qualitative) is much more relevant in informing such decisions. Ultimately though, domain expertise matters more than anything else as you’re fundamentally betting on creating a significantly different future than the one that exists today and that requires unique insight and perspective. It’s also why intuition matters here more than in any other category of high-stakes decision as the act of synthesizing different disparate signals into a coherent thesis and actionable agenda is incredible difficult to do. This dynamic helps to explain why Salesforce and Snowflake were founded by ex-Oracle execs, Zoom was founded by an ex-Webex exec and why Workday was founded by an exec PeopleSoft exec. It’s also why the cost of advice for this category of decision is exorbitantly high and more than that of any other category of decisions.
Product strategy decisions are another interesting and unique category of decisions. They are a derivative of corporate strategy and core operational decisions inheriting elements of both. That makes it interesting because those two are somewhat orthogonal and dichotomous. For high growth technology orgs, product strategy is very closely intertwined with corporate strategy and is a mechanism to achieve it mostly via the ‘build’ path. The ideal approach to this category of decisions is probably the most heavily debated with incredibly diverging viewpoints on the subject in the valley. There is the Steve Jobs / Henry Ford viewpoint which is often encapsulated by the following Ford quote: “if I had asked customers what they wanted, they would have said a faster horse”. This viewpoint essentially puts heavy emphasis on domain insight driven intuition for decisioning. On the opposite end is the ‘lean startup’ viewpoint which makes the product discovery and development process highly iterative and almost formulaic. What I concluded during our experience which included launching 5 significant products, some of which did better than others, is that the truth is in the middle. The most successful products tend to have two essential foundations. These include 1) a value proposition based on unique customer (or user) insights, 2) unique technical underpinnings. The former tends to be more outside-in and the latter more inside-out. When these two things converge, magic tends to happen. Alteryx Designer as a product is the ultimate example of these two elements converging well ahead of these ideas becoming mainstream. Like corporate strategy decisions, there is a fair degree of judgement and intuition involved in product strategy decisions, however, rapid short-cycle experimentation can help dramatically improve and mitigate erroneous assumptions.
In conclusion, I’ll reiterate the following. High-stakes decisions in different domain spaces involve different considerations. No one has a crystal ball and no amount of evidence or data can eliminate the uncertainty from what is at the end of the day a probabilistic outcome with major consequences. That said, while no one bats at a 1.000, exceptional leaders tend to have significantly above average batting averages around achieving their desired outcomes from their decisions. From what I saw and concluded from this journey, this phenomena is not entirely due to luck. These folks tend to possess a high degree of intelligence, significant amounts of intellectual honesty, a healthy dose of humility, substantial self and situational awareness and exceptional emotional discipline. They tend to recognize that the hand they’ve been dealt is what they must work with and aim for outcomes within the bounds of what that hand can deliver. They recognize that if data is king then context is God. My hope is that this model can help aspiring business leaders hone their skills around improving their odds of achieving their desired outcomes from consequential decisions.
If you’d like to read specific case studies about how we handled various domain specific business decisions, I’d recommend checking out some of my other posts.