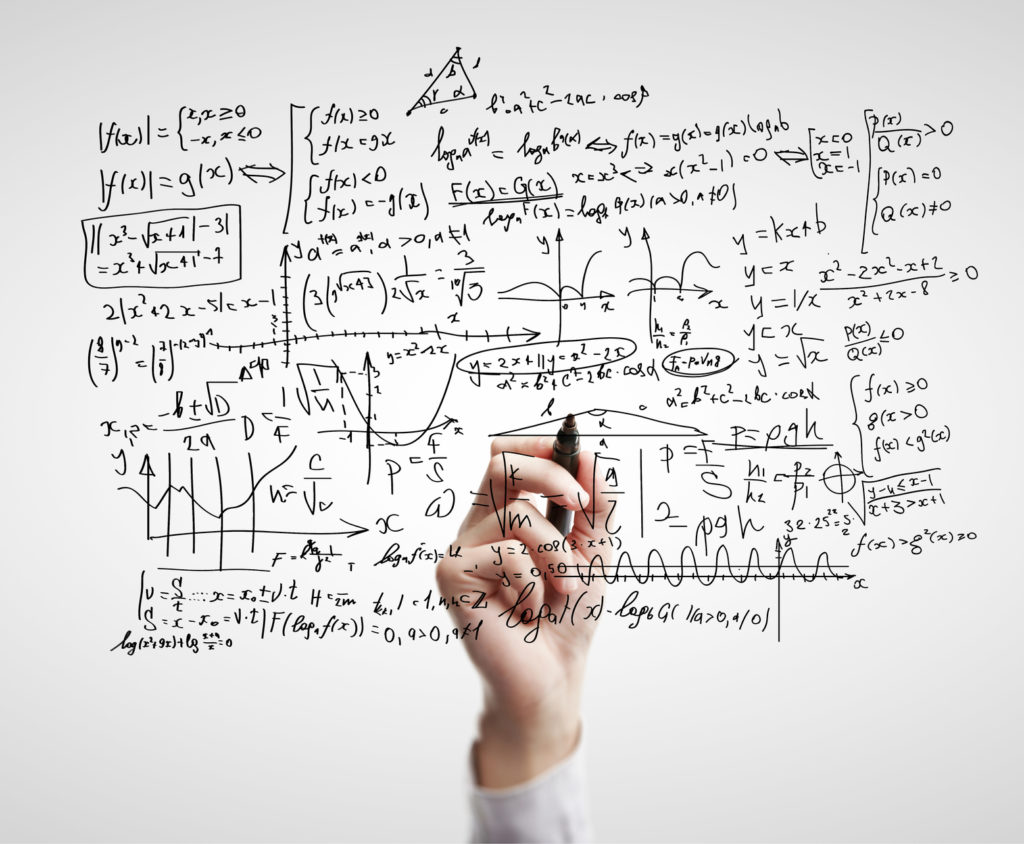
Predictive modeling use-cases can be separated into 2 broad types:
- Those where prediction itself is the prize. Accuracy tends to be paramount for such uses-cases and directly proportional to the value of the model (e.g. computer vision, direct mail targeting, high-frequency trading, lift modeling etc.)
- Those where insights into the relationship between predictor and response variables is the ultimate prize as it allows more effective decision making with regards to how to tradeoff and manage the prioritization of input variables (e.g. assessing the impact of remote working policies on productivity or the impact of education versus other factors on employee success)
Modern machine learning methods which are primarily non-parametric and rely on complex ensemble techniques are very well suited to the first task. Recent developments in such techniques have resulted in breakthrough advancements in predictive accuracy for seminal problems such as image and speech recognition. These methods however come with a serious drawback, namely the lack of interpretability and explainability in the relationship between predictor and response variables and the marginal effect each variable has on the outcome. This is why such contemporary methods are not well suited to the second class of problems described above. Relying on traditional parametric tools, which too have advanced recently, (e.g. regularization vs. standard regression) is a better approach to handling the second class of problems.
Organizational Implications for pursuing the different types of use-cases:
It is essential for an organization looking to utilize predictive modeling as a decision aid to determine what category their use-case falls into as that will dictate the structure of the teams and set of tools that are most suited to solve the problem:
- If it is a type 1 problem (which tend to be narrowly focused and very well defined) , then by all means, bring on the machine learning experts and let them have at the problem with all the data available. Hold out a test set of outcomes and evaluate each effort at solving the problem through out-of-sample accuracy in predicting this test set. You can then take the most accurate model and put it into production as all you care about is the model’s prediction (e.g. issue loan or don’t issue loan, what customers to target in a long list of candidates etc.). The model itself and its predictions can be embedded with relative ease into the workflow of managers who oversee the process (e.g. direct mail managers). These managers, for example, don’t really care “how” the 20 target customers were identified out of a list of 100. What they care about is the fact that these 20 are most likely to purchase a product when asked. Crowdsourcing platforms (e.g. Kaggle, KD Nuggets etc.) are worth considering for helping solve such problems as domain expertise is less relevant
- If the problem at hand can be classified as type 2 where insight about the marginal effect in correlation or the relationship between predictor and response variables is the prize (e.g. how performance in various attributes of a product results in the greatest variable margin over time or how various remote working arrangements impact employee productivity), then a different and more cautious approach is necessary as prediction accuracy in and of itself doesn’t create value. Classic econometricians, statisticians and domain experts with their traditional parametric techniques and hypothesis based approaches are likely to be more suited to the task as opposed to contemporary machine learnings experts who take a ‘data-out’ approach to the problem using non-parametric algorithms. The results and story they come up with has to be assessed for its business logic as much as its out-of-sample predictive accuracy. Identifying random and obscure correlations which is a benefit for type 1 use cases, can be a hindrance for type 2 use cases. Typically, such use-cases need to be refreshed on a less frequent cycle and insights from uncovering these relationships result in conversations that require a cross-functional set of middle managers to do things differently and possibly counterintuitively. Changing behavior is hard, which is why the explanatory aspect of the model is particularly important. Telling folks to diverge from established norms, convincing them of a change in organizational policy or requesting them to build a product differently because a “black box” said so is not a recipe for success
Contemporary machine learning and predictive modeling techniques have been hailed as a panacea by consultancies and the business press resulting in heavy investments by large organizations in building this capability. To be successful in using the power of these methods to create value, organizations must not treat machine learning as a silver bullet and instead be more thoughtful in assessing where ML can add value versus where traditional analytical techniques can be more useful. Yes, multi-layer convolutional neural networks are a breakthrough machine learning technology that will underpin machine vision in autonomous vehicles, however, they aren’t a form of artificial general intelligence and aren’t well suited to, for example, helping optimize capital allocation decisions in organizations. A discrete scenario analysis or parametric model is probably better suited for such a problem.
Tags: machine learning - predictive analytics